Entropy is a very important concept in physics. When I was taught about entropy it was always related to probabilities, and the problem sheets we did often featured betting, horse races, greyhounds, etc. All this came back to me this weekend with the running of the Grand National.
It turns out that bookmakers are using entropy when they decide their odds, albeit unknowingly.
Imagine a greyhound race in which there are six dogs running. The bookmaker assigns each dog an equal probability of winning – one-sixth – and thus each dog has odds of six to one (6-1).
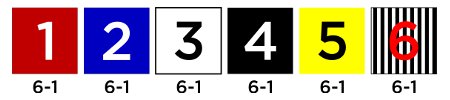
The problem for the bookmaker is that I can come along and bet an equal sum of money on each dog and be guaranteed to recoup my total bet, the bookmaker cannot make any money.
With longer odds the problem is even worse:
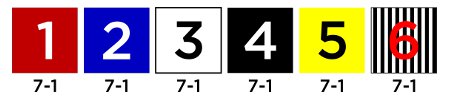
Now the bookmaker is guaranteed to lose money. A £1 bet on each dog for a total of £6 yields a win of £7 no matter what the result.
The obvious answer is to shorten the odds:
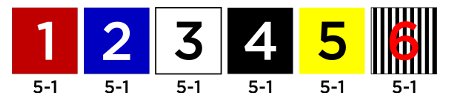
Now the bookmaker is guaranteed to make money, provided that all bets are evenly distributed amongst the dogs. If I bet £1 on each dog again, I can only win £5 and the bookmaker is guaranteed a £1 profit.
But this isn’t realistic: in real life there is usually a ‘favourite’, a dog generally considered more likely to win than the others. Therefore the bookmaker has to offer shorter odds on this dog, in order to avoid losing too much money and the opposite is true for the dog least least likely to win – the bookmaker can offer longer odds because he’s less likely to have to pay out.
But how can a bookmaker be sure that his odds have been calculated correctly? How can he be sure that, no matter what the outcome, he doesn’t end up too much out of pocket?
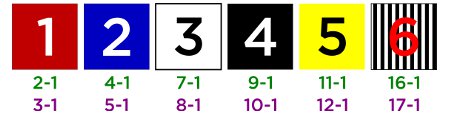
Which set of odds should he offer? The green or the purple? The green will result in paying out less money, but the purple will entice more customers to place a bet.
Unconciously, the bookmaker is calculating the entropy of the system. The more disordered the system, the greater its entropy. The greater its entropy, the greater the reward for the bookmaker. With six dogs each at odds of 6-1 the entropy is exactly 1. With the dogs at 5-1 the entropy is greater than 1; and at 7-1 the entropy is less than 1.
If we make a number of assumptions about how people bet, we can analyse the odds and calculate whether or not the bookmaker will make a profit.
- A dog’s odds are related to its chance of winning.
- Bets will be distributed amongst dogs according to their odds (e.g. people are more likely to bet on the favourite).
- With longer odds, more people will bet.
The green odds yield an entropy of 1.157 and the purple odds yield an entropy of 0.900. Assuming each person bets £1 and that 49 people will bet on the green odds and 55 on the purple odds the bookmaker can expect to make $6.66 on the green odds and lose £6.08 on the purple odds.
You can check yourself using the downloadable odds calculator (23.5kB, Excel). Change the bold values to check the outcome.
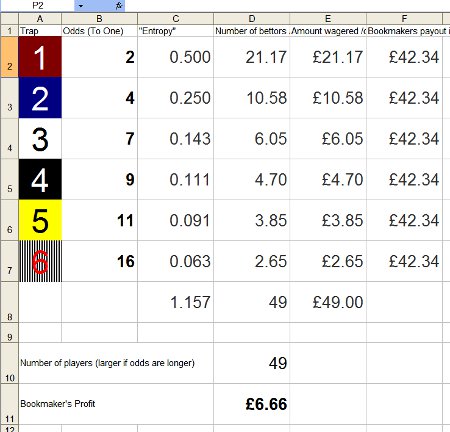
I worked it out and the Grand National’s entropy was 1.398, so Bookmakers should be happy!